Decisions are about the uncertain future. Our understanding of the future dictates the quality of our decision. The more we know about the uncertainty, the better is our decision. Hence the expression: “informed decision“.
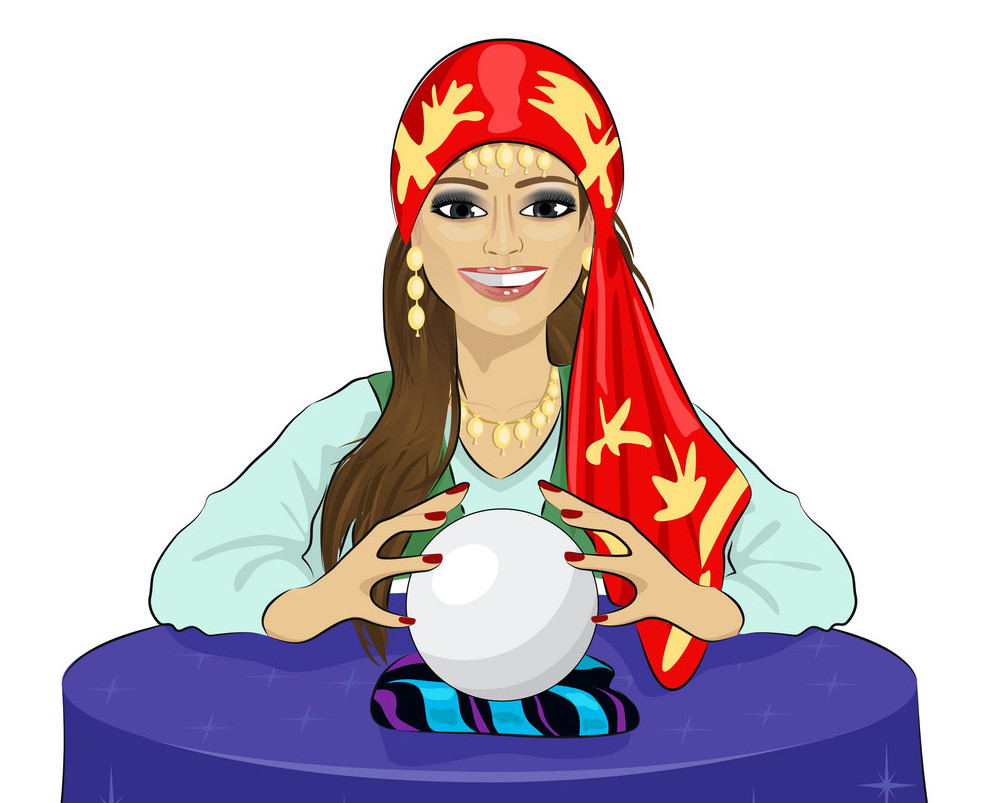
Additional Information about uncertain future
The question is how do we become “informed“? Well, since the future hasn’t happened yet, we try to collect information about another related event. The importance about this other event are two folded: 1) “related”. This event has demonstrated relationship with the uncertain future we’re interested in; and 2) the outcome of this related event can be observed before our decision, hence before the uncertain future realizes. In summary, in order to make a better decision, we collect information about a related event (let’s call it B) which would “inform” us about the uncertain future (let’s call it A). This idea of leveraging additional information B to understand the future A is Bayes Theorem.
The application of Bayes theorem in real-world context has two sequential stages, the induction (learning) stage and deduction (applying) stage.
II. Deduction stage
Let’s start with the stage we’re familiar with. The Deduction stage deals with the actual “informing”. We apply knowledge about the relationship between additional event B AND uncertain future A into using B to update A. Here are some examples of Deduction.
- Ex 1: When facing difficult decisions, we ask for other people’s advice (B) to update our understanding of the future (A).
- Ex 2: In email spam filtering, service provides use keywords (B) to identify spam emails (A).
- Ex3: In marketing, companies use small scale pilot study (B) to update the likelihood of success for a national launch (A).
- Ex 4: In business, companies use the report from consulting firms (B) to update perception of successful new products or projects (A).
- Ex 5: In healthcare, doctors use symptoms (B) to inform underlying illness (A). The list goes on and on.
However, one question remains, are all information (B) equally informative about the future (A)? Ex: Are advices from a trusted relative and that from a random person on the street equally useful? Are reports from various consulting firms equally reliable?
I. Induction stage
To answer that, we shift our attention to the less familiar induction stage. The Induction stage focuses on collecting historical data about the additional event B and the uncertain future A and on learning the relationship. Ex: yesterday morning it was cloudy (B) and then it rained (A). After observing many instances of the event (B) and future (A), we can induce how does the two relate to each other. The outcome of such induction is described as “reliability” or “track record” or “accuracy” of the additional information , which is in the form of conditional probability. More specifically, P(B|A) or P(Information | Future).
Here are some examples of Induction.
- Ex 1: In personal decision, if the situation was favorable, was the advice right or wrong?
- Ex 2: In email spam filtering, if the email was spam, whether the filter get it right or wrong?.
- Ex3: In marketing, if the national launch was successful, was the pilot study correct or wrong?
- Ex 4: In business, if the new products or projects was successful, was the report from consulting firms right or wrong?
- Ex 5: In healthcare, if the illness was caused by bacteria, was sore throat a good indicator?
To summarize, Bayes theorem is a process of inducing the reliability of additional information from historical data, and then update our understanding of the future with additional information. In the following video, we will look at an example together.